Artificial Intelligence (AI) is revolutionising industries worldwide, and the financial sector is no exception. From fraud detection and risk management to personalised customer experiences and trading algorithms, AI’s ability to analyse vast amounts of data, recognise patterns, and make decisions at incredible speed offers significant opportunities.
However, implementing AI in finance comes with its own set of challenges, particularly around data quality, governance, and ethical considerations. Without robust frameworks in place, AI’s potential benefits could be undermined by inaccurate predictions, biased outcomes, and reputational damage.
The Rise of AI in Finance
AI technologies are already driving innovation across the financial landscape. In investment banking, AI-powered algorithms can identify trading patterns and predict market movements faster than humans, giving firms a competitive edge.
In retail banking, AI personalises customer interactions through chatbots, tailored financial products, and AI-driven wealth management tools. Furthermore, AI is being deployed in credit scoring, loan approval processes, and even in reducing regulatory compliance burdens through RegTech solutions.
Despite these advances, the success of AI in the finance sector hinges on the quality of the underlying data and the robustness of the governance mechanisms overseeing its use.
The Importance of Data Quality
Data is the fuel that powers AI. In finance, where decisions have direct consequences on people’s financial well-being, it’s critical that this data is accurate, complete, and timely. High-quality data allows AI models to generate reliable outputs, which, in turn, drives better decision-making.
However, financial institutions often deal with massive amounts of unstructured and siloed data. The integration of disparate data sources, often containing inconsistent or inaccurate information, can introduce errors into AI models. Poor data quality leads to flawed predictions, which in turn can result in faulty credit risk assessments, failed anti-money laundering (AML) processes, or incorrect investment recommendations.
Speaking to Harnham at Big Data LDN last week, Seeta Halder, Head of Data Insights at Nottingham Building Society, said: “You can’t be artificially intelligent if you’re dumb with data.
“We were motivated to use AI early on, and one challenge we had while getting started was that some of our business functions were becoming quite siloed. We had inconsistent definitions, reporting into different committees… and by the time the data reached the board level we were seeing the same metrics with different numbers.
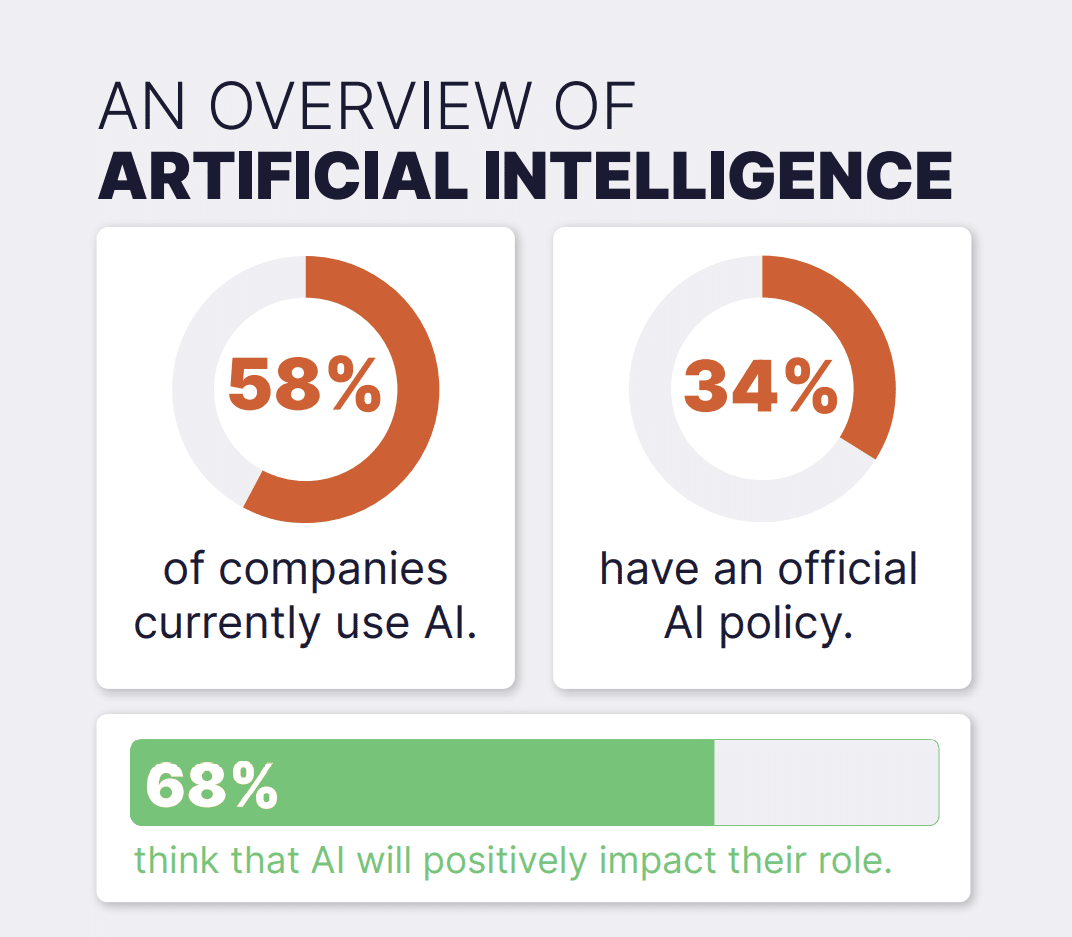
[AI is quickly becoming commonplace for modern companies. Source: Harnham]
Seeta went on to explain that data quality starts with proper data management practices, including continuous data cleansing, validation, and enrichment, as well as ensuring your team is receiving data upskilling and training. As financial data increasingly comes from a wide range of sources, including customer transactions, social media, and third-party data providers, maintaining high standards of data quality is essential to building trustworthy AI systems.
The Role of Governance in AI Implementation
Beyond data quality, governance is crucial for ensuring that AI is deployed ethically, transparently, and in compliance with regulations. In finance, where institutions are heavily regulated, a robust AI governance framework is necessary to mitigate risks related to bias, fairness, transparency, and accountability.
Martin Grant, Senior Product Manager at Barclays, told Harnham: “For me, it all comes back to trust. Do you trust your data? With all of the current regulations changing, do you trust your data to be compliant with those regulations? Do you trust the data that you’re sharing with stakeholders? You need to trust in that data, and only then can you truly use it with confidence.”
AI Governance in Finance
A strong governance model includes clear policies and procedures for managing the entire lifecycle of AI models, from development and testing to deployment and continuous monitoring. Key elements of AI governance in finance include:
- Bias and Fairness: AI algorithms are not immune to bias, which can arise from biased training data or improper model assumptions. In credit scoring, for instance, AI systems that rely on historical data may unintentionally discriminate against certain groups, reinforcing existing social inequalities. Governance frameworks must include procedures to detect and mitigate bias, ensuring that AI models promote fairness and inclusivity.
- Explainability and Transparency: In finance, decisions made by AI models must be explainable, especially in areas like lending, where regulators require institutions to provide customers with clear reasons for decisions such as loan approvals or rejections. “Black-box” AI models, which are difficult to interpret, pose significant challenges. Financial institutions need to implement explainable AI (XAI) techniques that allow stakeholders to understand and trust AI-driven decisions.
- Regulatory Compliance: Financial institutions are subject to a myriad of regulations designed to protect consumers, prevent fraud, and ensure the stability of the financial system. AI systems must comply with these regulations, including GDPR in Europe, which governs data privacy, and anti-money laundering (AML) laws. Strong governance helps ensure that AI models adhere to these regulations and that institutions can demonstrate compliance in case of audits or investigations.
- Continuous Monitoring and Validation: AI models in finance cannot be a “set-it-and-forget-it” solution. Markets and consumer behavior are constantly evolving, and models must be regularly monitored to ensure they remain accurate and effective over time. Governance structures should include mechanisms for continuous model validation, recalibration, and retraining based on new data and evolving circumstances.